The group pursues research at the forefront of physics and quantitative biology with emphasis on mathematical modelling and analysis of biological data, development of computational methods, systems biology, biophysics, biomathematics, artificial intelligence and general data science methods in these fields. We develop and apply predictive models for biological and biophysical systems and their interactions at multiple scales, and create statistical methods for the analysis of the complex correlated data. We are actively engaged in joint projects with experimental biologists and physicists producing such big data resources.
Much of our current research is directed for example at combining genomic sequence, expression level information and regulation network information with structural information such as high resolution microscopy and chromosomes conformation capture data to develop models to predict biological function. Our efforts have focused on the development and application of biophysical and bioinformatics methods aimed at understanding the structural and energetic origins of chromosome interactions to reveal the underlying physical folding principles. Our work includes fundamental theoretical research and applications to problems of biological importance as well as the development of appropriate software to handle the vast amount of data.
Areas of current activity include:
- The physics of chromosomes
- Relation between the gene network and the physical structure of chromosomes
- Physical models for gene regulation
- Modelling of cell regulatory networks
- Modelling of the synaptonemal complex
- Genomics and proteomics
- Modelling of tumor growth
- Bio-statistics
- Data science methods
- Image analysis
Research Partners
- Swammerdam Inst. for Life Sciences, BioCentrum Amsterdam, University of Amsterdam
- Shanghai Center for Bioinformation Technology (SCBIT), Shanghai, China
- CNRS, Montpellier
- Yale University, New Haven, USA
- Department of Physics, Boston University
- Instituto de Fisica, Universidade Federal Fluminese, Brasil
- German Cancer Research Center (DKFZ), Heidelberg
- Heidelberg Collaboratory for Image Processing (HCI), Heidelberg
- Leiden University
- California Institute of Technology
The group has access to state-of-the-art computing facilities which we use to perform our research and offers a broad scope of educational opportunities for graduate students working in the group.
Modelling of the 30nm Fiber (Chromatin)
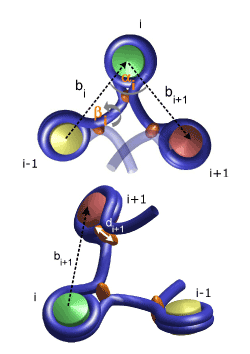
Modelling of the Chromosome in Interphase
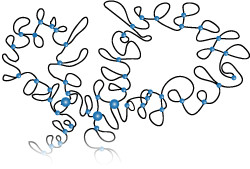
Modelling of gene silencing (in collaboration with the group at Montpellier)
Gene silencing refers to shutting down the activity (possibly temporal) of specific genes. Temporal gene expression data is of particular interest to biologists and physicists as it represents gene expression level within a biological system over time thus modelling the living system more accurately and closely as a dynamic living system. Classical modelling on the molecular scale as well as machine learning approaches are now increasingly being used for reverse engineering gene networks from gene expression data. We develop classical models as well as hidden markov models to understand gene silencing.
Development of patterns of coarse-graining and dynamical simulations methods with application to the mechanics of the genome structure in early G1 and co-polymers
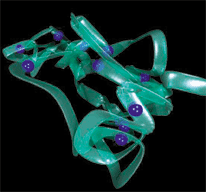
Mitosis is the process that facilitates the equal partitioning of replicated chromosomes into two identical groups. Before partitioning can occur, the chromosomes must become aligned so that the separation process can occur in an orderly fashion. The alignment of replicated chromosomes and their separation into two groups is a process that can be observed in virtually all eukaryotic cells. Specifically, we focus on the chromatin dynamics in the interphase which encompasses stages G1, S, and G2 of the cell cycle. We develop coarse-grained models under the perspective of a systematic coarse-graining pattern. From our previous work it has become apparent that much can be learned from studies of technical polymers. Hence, we want to investigate how we can identify and integrate out irrelevant degrees of freedom by studying co-polymers (both technical and biological).
Signal Transduction Networks in Cells (in collaboration with the group of Prof. Eils)
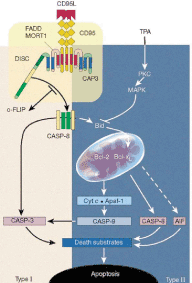
Agent-based modelling simulations in biology
Nonlinear models in physics and biology can exhibit a number of features not known from linear ones: a particular example is chaos and the occurrence of higher level features which were not explicitly modeled. Alas, these models can in general not be solved analytically and one needs to attack them by computer simulation. In some sense we want to create artificial live by modelling our systems via agents. These agents act as independent entities that carry needs and behaviour among other characteristics as well as a model of the world (environment). Due to their interaction patterns emerge that reflect certain aspects of the real world. We study these to look for critical phenomena in these and to understand the parameter dependence of these.